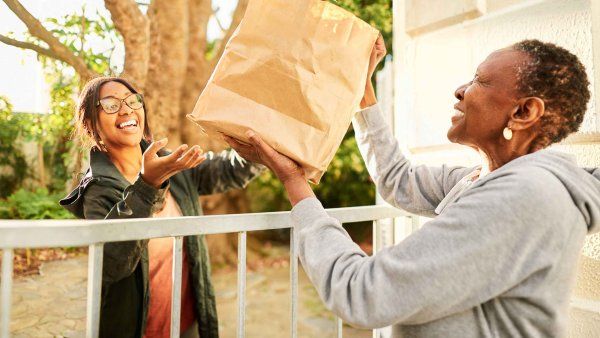
Imagine for a moment that we could create a model of the human brain so precise, so accurate, that it could mimic the brain’s intricate neural patterns in real time. Imagine a “silicon brain,” an artificial neural network so advanced that it could decode a human’s thoughts, restore speech to those who have lost it, and – perhaps one day – even generate a personalized model of the unique brain activity of any individual.
This isn’t science fiction. It’s the future I’m helping to build.
My lifelong fascination with the brain’s complexity sparked my interest in the intersection of neuroscience and artificial intelligence. I was drawn to the idea that AI can not only analyze massive amounts of data or help us write our emails or tell us which stocks to buy but also potentially mimic the fundamental aspects of being human – our ability to think, speak, and interact. In 2023, I joined the lab of Edward Chang, MD, at UC San Francisco to study brain-wide networks and even the activity of individual neurons in order to understand how our brains achieve a fundamentally human trait: language.
I feel incredibly fortunate to have entered this field when I did. For a long time, progress in neuroscience was slow because we didn’t have the right technology. Then, in the 1980s and 1990s, technologies for collecting brain measurements revolutionized the field. And now, particularly in the Chang lab, we have the ability to record the activity of single neurons in a person’s brain while they are undergoing brain surgery. Just 10 years ago, we didn’t think this was possible, but now we can track the activity of hundreds of single neurons, which is incredibly exciting. This will potentially revolutionize our understanding of brain circuits, especially those responsible for complex and uniquely human behaviors like language.
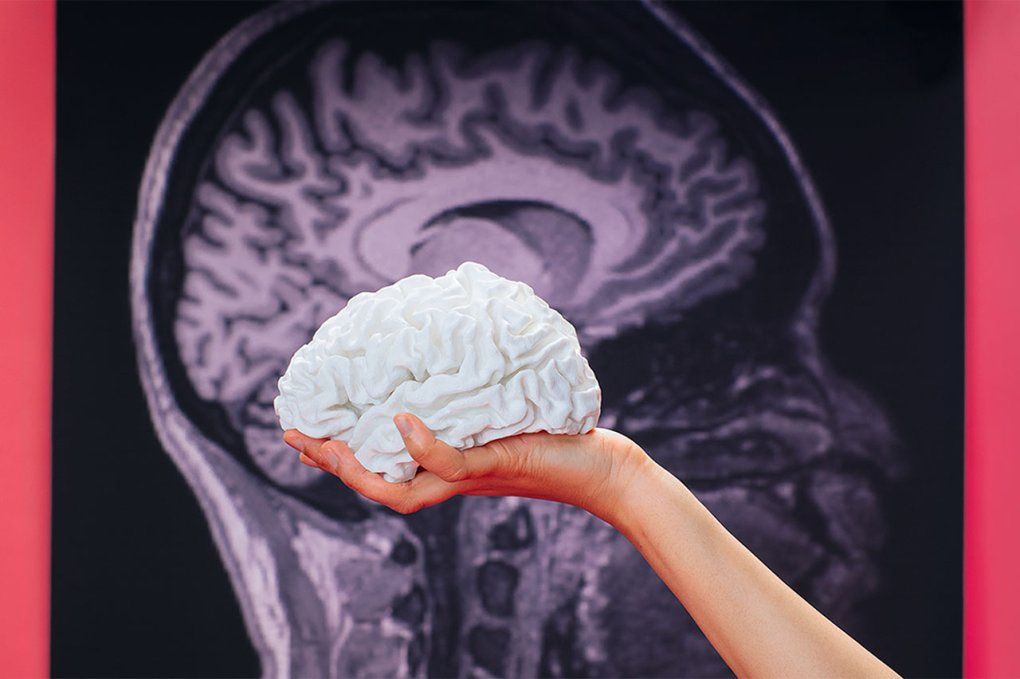
While this data is precious, to fully harness it, we also need powerful computational tools. This is where the recent surge in artificial intelligence and my background as a computer scientist come in. I’m hopeful that the fusion of AI and these new methods of measuring brain activity will transform neuroscience.
We are at a tipping point where AI’s capabilities, driven by vast amounts of data and powerful computers, are unlocking new possibilities to understand the human brain. Over the past decade, we’ve successfully used AI to build models of brain data collected with various imaging technologies, including functional magnetic resonance imaging (fMRI), magnetoencephalography, and electroencephalography. These technologies have been instrumental in advancing our knowledge, but we are only now starting to experiment with AI-based models of single neurons in the human brain.
At UCSF, we have the singular opportunity to collect amazing, high-quality, and diverse types of brain data through our neurosurgeons, neurologists, and psychiatrists. For example, fMRI systems can tell us which parts of the brain are active in a patient at any given moment, and diffusion tensor imaging tells us how different regions of the brain are connected to each other. And thanks to a very recent technology unique to UCSF, called neuropixel probes, we can even collect data from individual neurons. Eddie Chang – my boss and the chair of neurosurgery at UCSF – pioneered the use of these probes in humans in a series of revolutionary brain surgeries in recent years. During these procedures, the patient is awake and performing different tasks in the operating room while the probe records how individual neurons in their brain are firing. Observing brain activity at this infinitesimal scale is almost unimaginable. It offers a never-before-seen glimpse into the brain’s inner workings.
So in my work, I’m taking all these diverse sources of data and putting them into an artificial neural network. The goal is to produce the same patterns of “brain” activity in my artificial network that the patient’s brain produces.
The big challenge in my work is integrating all the data at our disposal into a single, working model. For example, fMRI is not a direct measure of neural activity but of how much oxygen different parts of the brain are using. The results tell us what’s happening across hundreds of thousands of neurons. It’s a very coarse measurement, but it is valuable for seeing patterns of activity across the entire brain. On the other end of the spectrum, neuropixels give us extremely high-resolution data from individual neurons, but we don’t get a full-brain picture. I am trying to create AI models that can integrate the best of all worlds. By building models that process many different types of data modalities, we hope to develop a fuller picture of the human brain.
But we’re looking not just at neural data; we’re also incorporating text inputs that a patient might read or hear; the speech the patient produces or listens to; and behavioral data, such as how well the patient understands a specific sentence or can solve a math problem. By combining all these data sources into the same artificial neural network, we can create a “silicon brain” – a model that can produce the same patterns of brain activity as a human brain.
One of the most exciting applications of this technology is in the development of new generations of brain-computer interfaces (BCIs). In recent years, Dr. Chang and his team have used BCIs to restore the ability to communicate to individuals who are paralyzed and can’t speak. But while current BCI systems are powerful, they must also be highly personalized, requiring extensive training data from each new patient to function effectively.
This is where the concept of a silicon brain comes into play. By training an artificial neural network on vast amounts of neural data from many different people, I believe we can create a model that doesn’t work just for one person but could be adapted to work “out of the box” for any patient. Imagine a device that could be deployed in any patient without the need for extensive calibration – a device that could restore speech or movement from day one. The implications for patient care are enormous.
Designing a Digital Twin
Roll over a + to learn more.
Illustration: Farah Hamade
Designing a Digital Twin
Click on a + to learn more.
Immediate Speech Restoration
Develop a device to help patients speak again without needing extensive adjustments for each person.
Tailored Mental Health Treatments
Create individualized treatment plans for conditions like bipolar disorder, schizophrenia, and depression.
Outcome Predicitions
Use a patient’s silicon brain model to predict surgical outcomes.
Illustration: Farah Hamade
In the realm of mental health, the applications of this technology could be even more profound. For too long, our understanding of neuropsychiatric conditions has been limited by the tools at our disposal. Conditions like schizophrenia, bipolar disorder, and depression are incredibly complex, involving multiple regions of the brain and intricate networks of neurons. While we can’t dissect a living human brain to see how its components work, we could do this with an artificial brain model.
By feeding an AI system with data from patients with specific neuropsychiatric conditions, we could start to see patterns in how different parts of their brains interact and how these interactions may go awry. This could lead to new, more targeted treatments that address underlying neural mechanisms rather than just alleviating symptoms.
And as we move closer to simulating different brain disorders within an artificial model, we will be able to conduct experiments that would be impossible in a human patient. We can explore how certain stimuli or interventions affect neural activity in a controlled environment, allowing us to better understand and treat these conditions. In time, we could expand on this to study how the brain perceives the external world, retrieves memories, and ultimately produces thought.
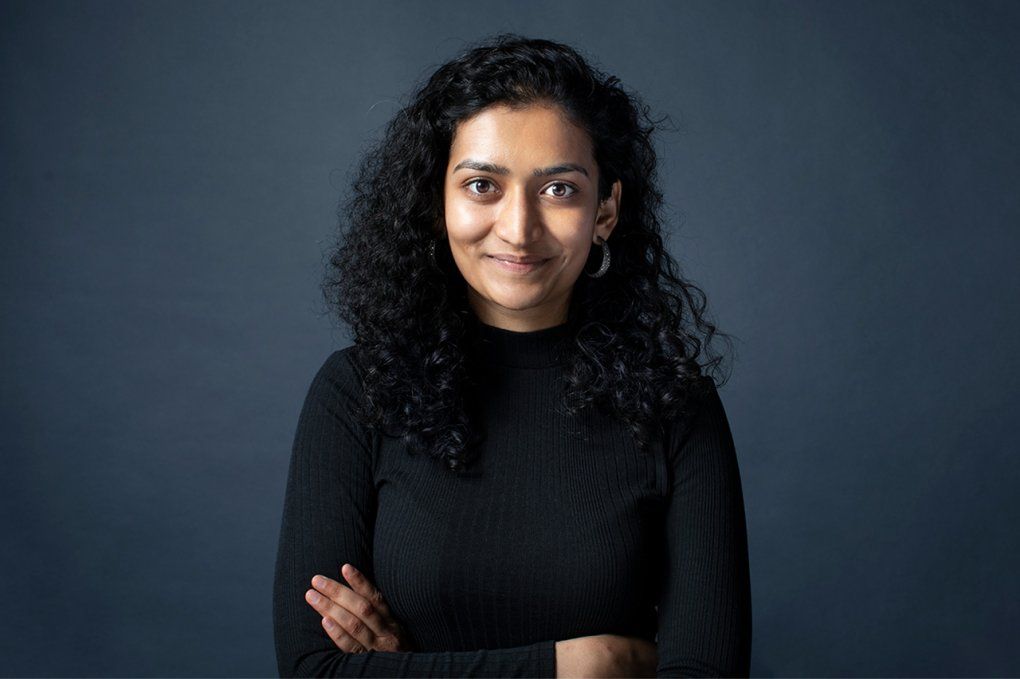
Looking 20 to 50 years ahead, I believe an AI system trained on brain data could create a “digital twin” of an individual human brain. These AI-generated models could replicate not only general brain activity but also the specific neural patterns of an individual, enabling personalized insights into how that person’s brain functions. Before undergoing brain surgery, for example, a patient’s digital twin could be used to simulate the procedure and predict its outcomes. Or a clinician could build highly individualized treatment plans for neuropsychiatric conditions based on each patient’s unique brain activity patterns.
We are still in the early stages of all this, and the journey ahead will involve not only refining these models but also ensuring that they are applied ethically and equitably in clinical settings.
There are huge ethical questions involved, especially when it comes to consent and data privacy. When working with human brain data, ensuring that participants fully understand what their data will be used for is crucial. We also have to consider the potential for misuse of the technology, especially if these models become advanced enough to predict individual brain activity. As these models become more mature, we’ll need to have more conversations about ethical implications. We can start now by educating people about what these models are and what they are capable of.
These are provocative ideas. But technology is progressing quickly, and we might be closer to the future that I’m describing than we realize. By harnessing the power of AI, we are not just imagining the future – we are building it.